Features
Automate personalization, optimization and testing across the user journey with a full suite of tools. You’ll be on your way to increasing cart values, conversion rates, return visits, and whatever KPIs you’ve set out to conquer.
See how trbo works on your site
Personalization
Create exceptional, unique customer experiences to drive growth.
- Adapt your existing site content to your customers - automatically.
- Greet your return customers with a personalized message.
- Welcome new users with a custom message based on 50+ identifiable characteristics.
- Incentivize returning visitors with relevant offers and promotions.
Audience Segmentation
Identify and learn about your site visitors for better targeting.
- Set up an unlimited number of audience segments.
- Define audience segments based on user interests, preferences, and behavior - even when these factors change.
- Easily integrate on-site and off-site data.
- Create rule-based or dynamic AI-powered audience segments.
- Easily view all your audience segments in the trbo dashboard.
Recommendations
Maximize every customer touchpoint the second they land on any of your channels.
- Predict and deliver the most relevant brand experiences with cohesive messaging, products, and offers.
- Trigger recommendations based on specific behaviors, events, schedules, referral links, and interests.
- Select experience and interaction templates from our large and ever-growing library.
- Deliver real-time product and promotional recommendations across all your channels.
- Reach customers at every point in their journey — no matter the device.
Analysis & Optimization
Stay in the know to consistently improve the customer experience on your website.
- Collect 50+ on-site visitor characteristics automatically with trbo.
- Connect and leverage your business’ entire marketing tech stack, including your email service provider, CRM, and much more.
- View all your data and performance in customizable dashboards.
- Quickly uncover insights to take actionable steps towards optimizing your website.
- Easily share reports and collaborate with team members.
- Work with trbo’s team of experts to identify opportunities for optimization.
A/B & Multivariant Testing
Test hypotheses and increase KPIs.
- Craft intentional experiments for every stage of the consumer journey.
- Learn with (dynamic) A/B testing, multivariant testing and multi-armed bandit testing.
- Quickly see results and make better decisions to optimize your customer journeys.
Customer Data Enrichment
Leverage and enrich your customer data.
- trbo captures over 50 visitor characteristics for AI-powered personalization.
- Enrich customer data with interfaces to CDPs, BI solutions and other systems.
- Implement a highly personalized approach with a 360-degree view of your customers.
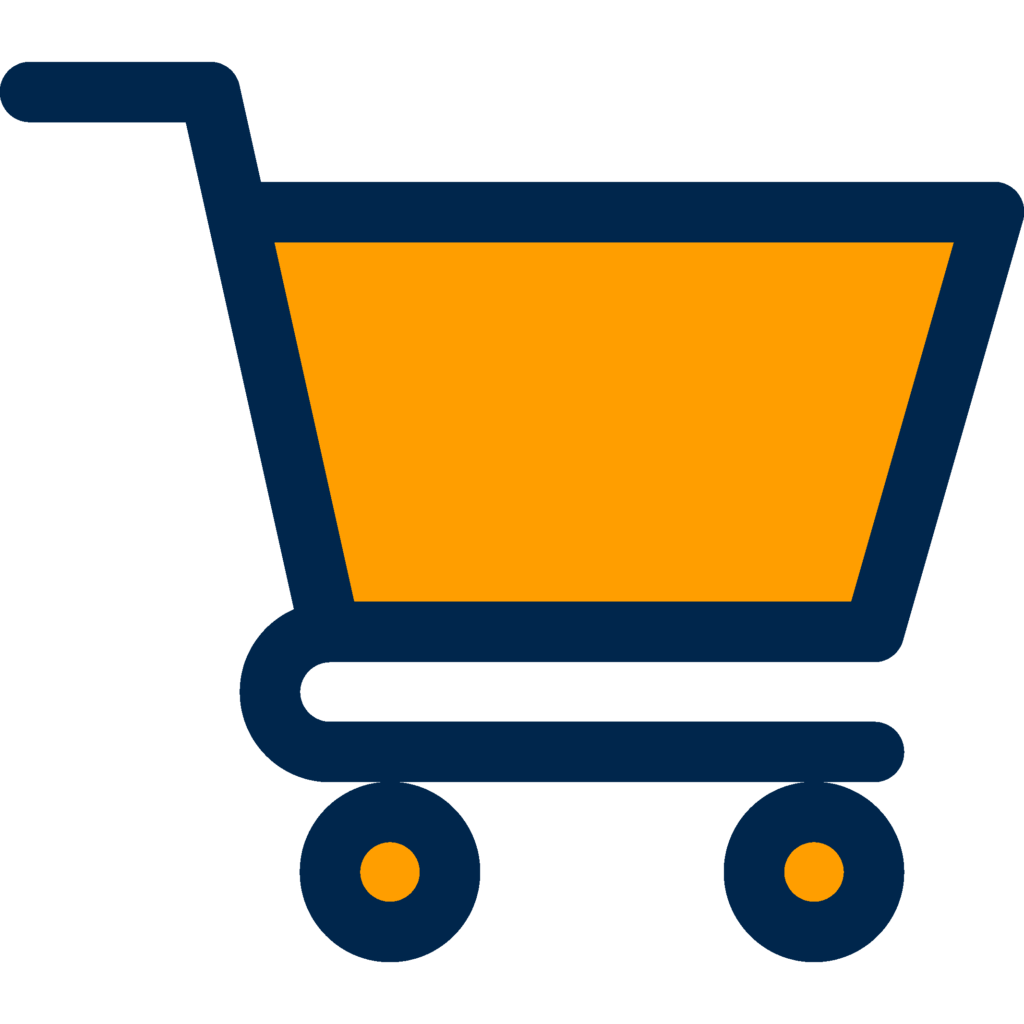
Product Advisor
Guided Selling for a perfect shopping experience.
- Completely integrated full-screen advisor.
- Visual product advice and inspiration in theme worlds.
- Step-by-step consultation process.
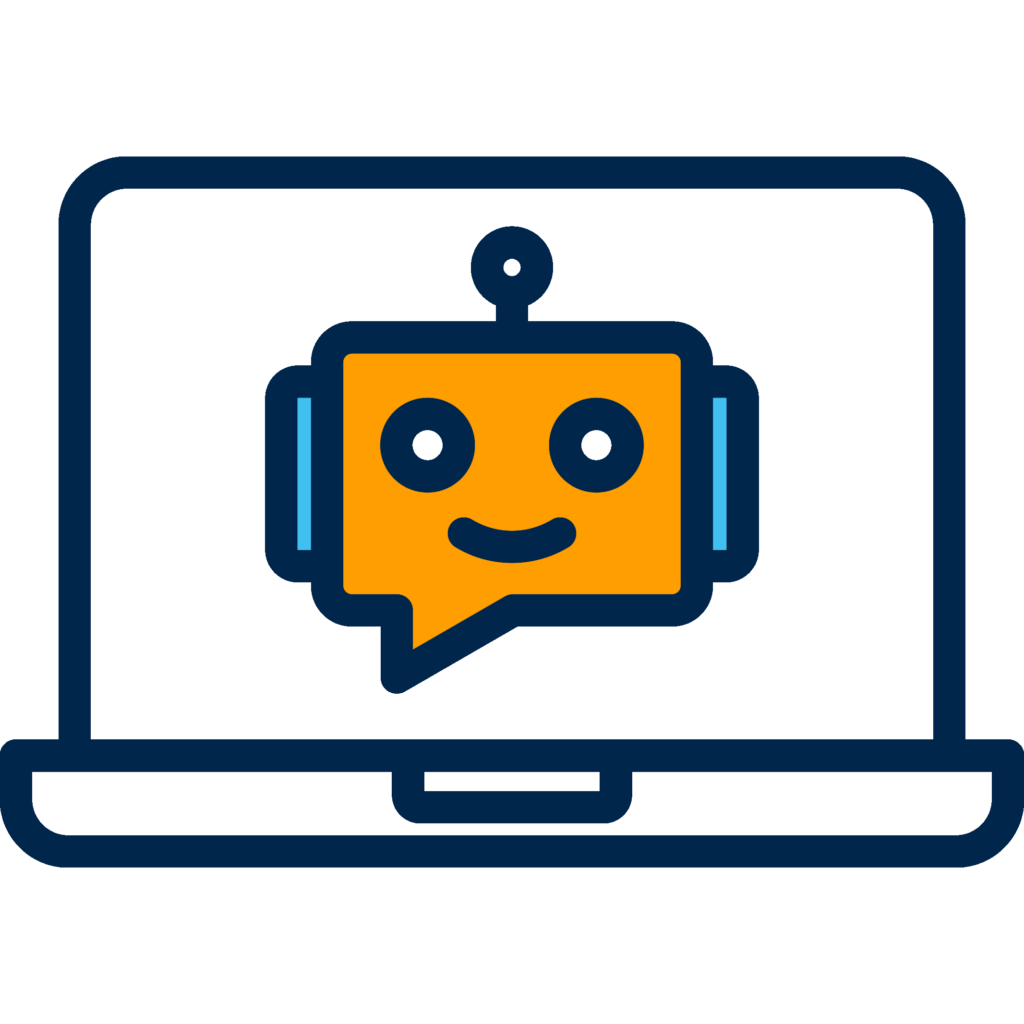
Shopping Assistant
The perfect digital product advisor for your online store.
- Interactive product advice via chatbot.
- Fits seamlessly into your webshop with custom designs.
- Matching product recommendations based on your customer's interests.
- Customer journey extension with lead generation.
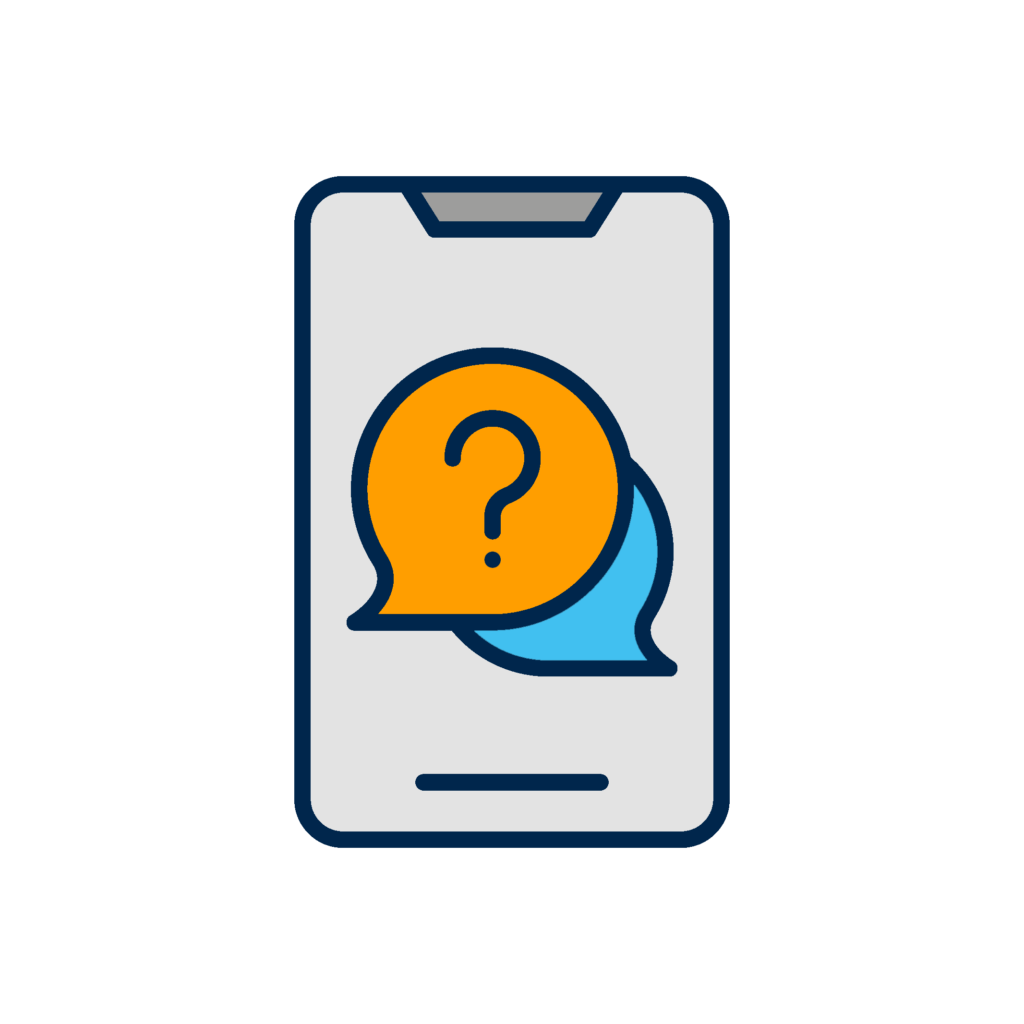
Chatbot AI
Reduce the workload of your customer service team.
- Powered by ChatGPT (OpenAI).
- Automate recurring customer inquiries.
- Training based on existing FAQs, essential knowledge from your customer care team as well as website content.
- Seamless integration with various store systems.
Personalized Emails & Triggers
Take your email marketing to the next level.
- Optimize newsletter subscriptions.
- Generate sales with the right email triggers.
- Increase KPIs with personalized recommendations.
Surveys & NPS®
Unlock customer insights.
- Measure loyalty and advocacy with NPS®.
- Use insights to optimize your website.
- Enhance customer satisfaction.
Gamification
Boost user engagement and conversions with gamification.
- Multiple options for gamification campaigns available.
- Interactive experiences with your website.
- Motivate your customers with rewards.
Discounts & Vouchers
Targeted offers instead of one-size-fits-all.
- Unlock the power of personalized savings.
- Dynamic discounts for every shopper.
- Rewarding loyalty, one voucher at a time.
- Re-engage and convert with targeted promotions.
Inspiration Pages
Enlighten your customers with Shop-the-Look inspiration pages.
- Simplify the user journey.
- Curate compelling visual impressions.
- Emotionalize the shopping experience.
- Automate and personalize the content curation.
Product
Bundling
Maximize sales with carefully selected product bundles.
- Unlock maximum sales potential.
- Simplify decision-making for customers.
- Experience seamless sales optimization with product bundles.
Don’t see what you’re looking for?
trbo has a lot in its toolbox – and we’re always working to stay ahead of the curve. Chat with one of our experts to discuss ideas for your site.
Works with all your current data partners, tools, and teams. We plug right in.
Partners and Interfaces
No more data silos. We augment all of your existing partners and external data sources to give you more power to make actionable decisions from insights on your customers.
Dedicated Expert Support
trbo is a self-service platform that gives you 24/7 access to your campaigns. You can create, edit, pause or optimize campaigns right from your device. Of course, our personalization experts are always there for you to answer questions and provide recommendations.